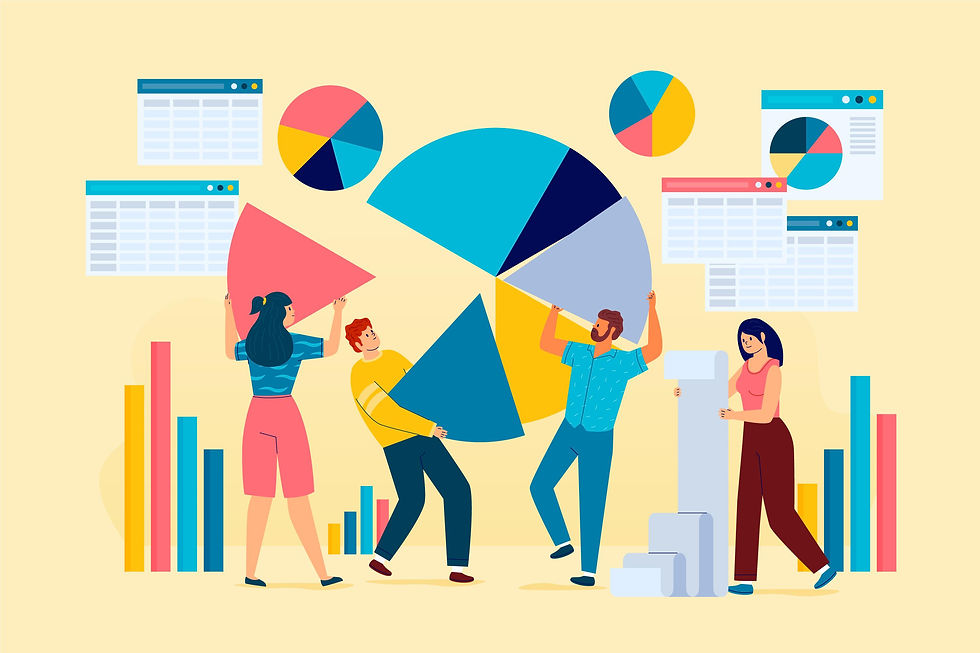
“Cohort Analysis” are,
Spending time building the right questions.
Choosing the right output and visualization for your audience and
Build a Cohort Analysis.
So, let’s get started!
Spending time building the right questions.
Asking the right questions form an integral part of building a cohort analysis because without the right question one cannot get an expected answer. After all, we don’t want to waste time or get to incorrect conclusions or have data that does not add value to any business… Don’t we?
That makes one wonder how to work out a meaningful question?
This is where our most commonly used SMART principle comes into the picture. Usually, the SMART encourages to define a given question or task or requirement to be Specific, Measurable, Actionable, Relevant and Timebound. But when it comes to Cohort Analysis, such a generic framework for defining an analytical question cannot get the “Spot on” details one might look for.
But, the same can be achieved by this customized framework (SAD), which makes sure your questions are
Segmented
Detailed and
Actionable
For instance, let’s see this question
“What percentage of users interacted with the recommended feature last month?”
Does it ring any bells here? There can be many types of users or many features launched within the application isn’t?
In order to be specific, let’s use the SAD framework to reword the same question.
What percentage of paying users interacted with the recommended feature last month? — Segmented
What percentage of paying users made at least one recommendation feature last month? — Detailed
What percentage of paying users made at least one recommendation feature last month compared to this month? — Actionable
Few more examples for your understanding…
One might also consider if the data we give to the customers is actually right! This is where experimentation adds validity!
Hypothesis testing is the most statistically reliable way to validate the results from feature changes. All you have to do here is to compare the results of two user groups, one who is exposed to the feature versus not exposed to the same. Collecting and comparing the user metrics via experimentation will help us to move forward to make reliable decisions.
Choose the right output format and help to visualize clearly
In the previous section, we looked at how to ask the right questions in order to track appropriate data or metrics. Now, we have to present the results in proper output formats with the help of some visualization to the intended audience.
The output can be presented based on what the audience asks for. It could be as simple as an email, SMS or a report, dashboard or a document in CSV/ PDF formats. Picking up the right format totally depends on
Frequency — Whether it is a one time or recurring activity.
Audience — Same data will be presented in a different manner to a sales team and a technical team.
External or Internal usage — To be circulated within internal or with external teams.
Privacy vs Transparency — Which audience has to know what level of information.
So far, we determined who requires what! Now let’s see how better you can present data to the intended users. Visualization plays a vital role because any badly presented data loses its value, in spite of covering all the proper metrics.
A few best practices you can follow while presenting a graph or a dashboard are,
Keep the graphs or plotting simple without colourful decorations. Unless needed to emphasize a particular point, reducing the use of bold colours will be pleasant to the eye.
Use chart types which helps user to compare data in literally a few seconds. For instance, a comparison chart when given in a line graph is more receptive to eyes than a bar graph.
Follow proper alignment when it comes to presenting numbers in the table.
Always choose the right chart type that is simple and conveys the best information to the audience.
Never distort the Y-Axis unnecessarily!
Once the initial data collection and validation is done, the next step is to perform the Cohort Analysis.
Cohort Analysis
A cohort is a group of people that are bound by one common trait. One can create cohorts based on any information or factors.
For instance,
No of users from Antarctica (Geographic segmentation)
No of users whose company size is from 1–10 employees (Demographics) are a few valid cohort groups.
But why Cohort Analysis??
To arrive until this point, one might have spent some precious time to get data by asking the right questions, validating it and finally putting the data in a presentable format. Is it even worth it??
The answer is Yes! All the hard work from your previous steps resonates here to study a few important metrics that can actually help you to improve your product/ business retention.
How to create one? It’s simple! Consider the below possible steps to categorize your data based on the following,
Once you are done with the above-mentioned activity, you can plot the data similar to the one below, study the details for each month and arrive at a possible conclusion.
You can find several templates on the internet for cohort analysis that helps you get hands-on in the concept and improve your learning.
References: Learnings from "Product Analytics 101" hosted by The Product Folks [Mathew Brandt]
Comments